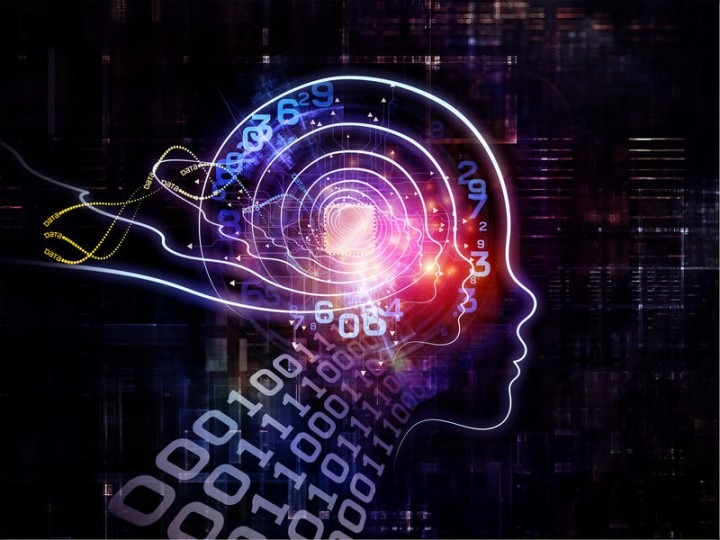
This development creates the possibility of using AI to help people with speech difficulties caused by conditions such as stroke or motor neurone disease
Researchers have developed an AI-based decoder that can translate brain activity into text without invasive measures for the first time. The decoder can reconstruct speech with high accuracy while someone listens to or imagines a story, using only fMRI scan data. Previous language decoding systems required surgical implants, but this breakthrough raises the possibility of non-invasive restoration of speech for people who have difficulty communicating due to conditions such as stroke or motor neurone disease.
The achievement has overcome a significant drawback of fMRI technology, which is that although it can accurately map brain activity to a particular area, there is an inherent delay that makes real-time tracking of activity impossible. This delay is due to fMRI scans measuring the blood flow response to brain activity, which takes about 10 seconds to peak and return to baseline. Even the most powerful scanner cannot improve on this lag. As Huth explained, this creates a “noisy, sluggish proxy for neural activity.” This limitation has made it challenging to interpret brain activity accurately in response to natural speech as it provides a “mishmash of information” spread over a few seconds.
To teach the decoder to match brain activity with meaning, three volunteers had to spend 16 hours each lying in a scanner and listening to podcasts. The decoder was trained using a large language model called GPT-1, which is a predecessor of ChatGPT. Afterward, the same participants were scanned while either listening to a new story or imagining telling one, and the decoder was used to produce text solely from brain activity. The text matched the intended meanings of the original words closely or precisely around half of the time.
An example of the decoder’s performance is when a participant heard the phrase “I don’t have my driver’s licence yet”, and the decoder translated it as “She has not even started to learn to drive yet”. In another instance, the phrase “I didn’t know whether to scream, cry or run away. Instead, I said: ‘Leave me alone!’” was decoded as “Started to scream and cry, and then she just said: ‘I told you to leave me alone”.
At times, the decoder made mistakes and faced difficulties with specific language elements such as pronouns. According to Huth, it could not distinguish between first-person or third-person, or the gender of the speaker. The reason for this weakness is unknown.
Moreover, since the decoder was tailored to each participant, it generated unreadable results when tested on a different individual. It was also possible for the trained participants to trick the system by thinking of animals or visualizing a different story quietly.
Professor Tim Behrens, a computational neuroscientist at the University of Oxford who was not part of the study, praised it as “technically impressive” and said that it creates several experimental opportunities, such as reading the thoughts of someone while they dream or examining how novel concepts emerge from background brain activity. “These generative models allow you to observe what is happening in the brain at a new level,” he said. “This means that you can truly extract a deep understanding from the fMRI data.”
The team aims to evaluate if the approach could be used with other portable brain-imaging systems, such as functional near-infrared spectroscopy.